Man and Machine: A Hybrid Approach to Athlete Load Management
- Jo Clubb
- Feb 15, 2023
- 6 min read
Updated: Aug 7, 2024
Man vs Machine: a debate fuelled by science fiction and Hollywood. As technology advances, the battle has garnered more and more attention…
In the red corner, Machine, armed with gigantic processing power that provides the ability to rapidly identify patterns or trends that may not be immediately apparent to humans. It does not tire, nor make silly mistakes purely due to “human error”.
Yet, the machine is reliant on their human operators. It is hampered by Garbage In Garbage Out and relies on the human operator to input suitable contextual factors.
In the blue corner, Man, armed with expert domain knowledge. Sentient beings equipped with the day-to-day experience managing athletes in reality.
Man benefits from cognitive biases that help make sense of the world, providing mental shortcuts to reduce cognitive processes. Yet, these shortcuts can be a double-edged sword. For example, the ‘affect heuristic’ enables us to rely on our emotions, but can sometimes hamper our decisions.
The us vs the machine storyline is of course appealing to fiction writers. But these concerns are somewhat shared throughout the sports science community. During my market research for this series on artificial intelligence (AI), I received comments such as…
“My main concern is that some practitioners might overestimate the intelligence of that AI and not question the data/interpretations. ‘Machine says so, it is very expensive/complex, so it should be truth!’”
“Nothing will outsmart human interaction, personal relationships & the human brain.”
Can we bring the machine into our corner and battle together against injury and underperformance?! Can we utilise its strengths, while being mindful of its limitations? Maybe a peace process is required. If so, the notion of how the human operator can appropriately consume and implement such information from a machine teammate is a critical conversation.
Training Load Management Decision Making
When it comes to load management, we spend a lot of time discussing the collection and analysis processes: the technologies, metrics, timeframes, calculations (Acute: Chronic Workload Ratio debate, anyone?!).
And yet, there appears to be less discussion about what we then DO with all that information.
So, before we even consider how AI might be integrated into that process, let’s take a step back and consider the broader load management decision making processes.
Contemporary training load philosophies build upon Banister’s fitness-fatigue theory (Banister et al., 1975). A training stimulus results in two antagonistic responses; a short-term negative effect (fatigue) as well as a longer-term positive effect (fitness).
With repeated training exposures, an optimal balance of fitness and fatigue must be achieved to maximise (physical) performance. Balancing an athlete’s training load with sufficient recovery and rest, aids in preventing excessive fatigue while also promoting adaptations and/or maintaining fitness.
This theory provides the foundation for load management decision making. Of course, we still have much to learn in this area. We need to better understand the causal pathways of injury and how the complex systems approach applies to sport.
In the applied setting however, we are responsible for applying current knowledge and technology to support decision making. So, what decisions are being made and what interventions are being employed?
Some fear a leap to pulling athletes out of training and games when it comes to load monitoring. How often does that actually happen? Is it reality or a widespread narrative that we are battling?
For me, pulling a player out of a game or even training on load monitoring grounds is the absolute last action taken. It would only occur if supported by significant injury concerns and/or notably poor response data. Plus the decision would have to be made in a collaborative, interdisciplinary manner.
More often, we are working from a wider menu of interventions. See Figure 1 below for a hierarchy of load management interventions.
Figure 1
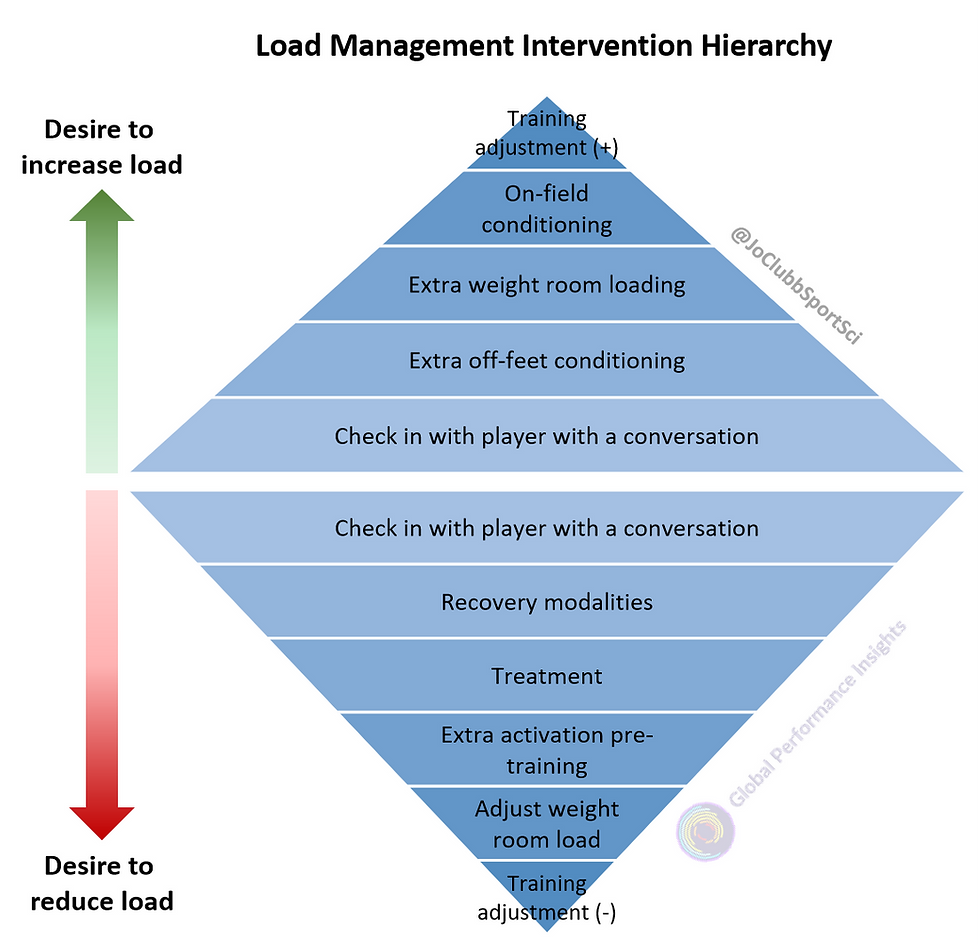
These interventions go in both directions; underloading and overloading. It is not just about reducing training load. There should be just as much debate about increasing workload. Some may shy away from this, as there seems to be a greater responsibility if something goes wrong. Yet, we know the benefits of building chronic workloads and physical capacities.
Man AND Machine: A Hybrid Approach
So, how might AI be integrated into this process?
As we discussed previously, the output design is crucial in guiding the user. AI applications outside of sports science may warrant a “go/no-go” decision. Yet, as Figure 1 demonstrates, there are many potential layers of intervention in load management. Providers should design interpretable and actionable outputs, but this remains far from making the final decision in place of the human operator.
In the book, The Signal and the Noise, Nate Silver talks extensively about a hybrid approach to decision making in sport. He advocates for scouts to use both intuition and algorithms.
“The fuel of any ranking system is information - and being able to look at both scouting and statistical information means that you have more fuel.” - Nate Silver, The Signal & the Noise
A hybrid approach is also suited to training load management. While constantly evolving, sports science data inputs are not all encompassing. And although we’ve discussed the importance of layering contextual information into analytics, there is a multitude of day-to-day context that is only visible to the practitioner. I suspect, regardless of AI, most teams and practitioners currently operate some form of hybrid approach, utilising a mixture of data and intuition to guide these decisions.
When it comes to the specifics of how to apply a hybrid approach, there is no one-size-fits-all approach. Rich Buchanan, Performance Director for Zone7, shared the following on how he has witnessed the integration of their system in applied practice.
How Zone7 Users Integrate a Hybrid Approach
Practitioners working in data-rich environments without an AI system are absolutely operating in a hybrid manner by conducting manual analysis of siloed datasets and cross referencing with expert human opinion. So, when introducing machine learning data analysis to this established hybrid approach, it is important for practitioners to fully understand what the added value really is.
A mistaken perspective I sometimes encounter in the industry, is one where there is a desire or expectation for AI to be definitive and flawless. To devolve responsibility to the machine, receive direction and instruction in pursuit of no false positives or false negative cases. This, however, is unrealistic in a radically uncertain environment that is sports performance.
Despite AI-derived insights being generated more efficiently, comprehensively and consistently compared to analogue methods, they still need to be cross-referenced with expert human knowledge. Once it is accepted that AI-derived insights are probabilistic and provide a positive contribution to a hybrid working model, then meaningful application and impact is far more likely.
Practitioners utilising Zone7 often find great comfort when the system reaffirms their own perspective, improving psychological certainty when determining the most appropriate course of action to take from the intervention hierarchy. A greater challenge for practitioners is when the system presents information that conflicts with their professional perspective. However, it is in this situation that I personally feel the greatest value is to be gained from tools like Zone7.
A scenario where man and machine have conflicting perspectives in the workload management and injury risk forecasting domains should be viewed as a safety net. At the very least, it should facilitate further discussion and consideration. Following further multidisciplinary discussion, it may change opinion and ultimately course of action. This is not a case of being data-driven by a machine, but simply man and machine operating as dependable teammates.
Dr Imtiaz Ahmad, Head of Medical at QPR FC, illustrated this for a recent masterclass:
“As with all computer generated perspectives, we do not blindly follow all Zone7’s recommendations. The way I see it is as part of our team. So, if I’ve got six or seven decision makers that work with me at QPR, I consider Zone7 as one of those as well. It is like having another member of staff – a member of staff that has got different opinions to me and comes to those [opinions] in different ways. Sometimes I like it, sometimes I don’t.”
What Next?
In this series on AI in sports science, we have journeyed through the entire data pipeline. We defined key terminology in our AI Dictionary, and then discussed the model inputs, the contextual layers, the need for transparency in analysis, and most recently, the output design decisions.
The final juncture of applying the data, discussed above, is critical. Even with suitable inputs, context, analysis, and outputs, load management decision making should not be relinquished to the machine altogether.
Sports performance, physiology, and injury are complex entities that we still have much to learn about. While the volume of data collected in these realms has rapidly evolved in recent times, there remain a multitude of gaps. Gaps in both our knowledge and our data.
A hybrid approach may benefit by combining the processing power and analytical capabilities of the Machine, with the experience and context of Man. Ultimately, such systems can provide perspective but it is the human operators who remain fundamental to the final decisions.
Thanks to everyone who has contributed to and read this series on AI in sports science. I’ve learned a lot but appreciate we still have a lot of learning to do!
This article is supported by Zone7. For more information about their technology, visit their website.
Comments